Within a few years, artificial intelligence went from science fiction to real-world usage. But how does AI function behind the buzzwords? If you’re wondering about how “artificial intelligence,” “deep learning,” and “neural net” interact or want to learn more about generative AI, you’ve come to the correct spot.
They will define “AI,” “machine learning,” “neural net,” and “deep learning” from a high level and work down. As seen above, these terms are hierarchically related:
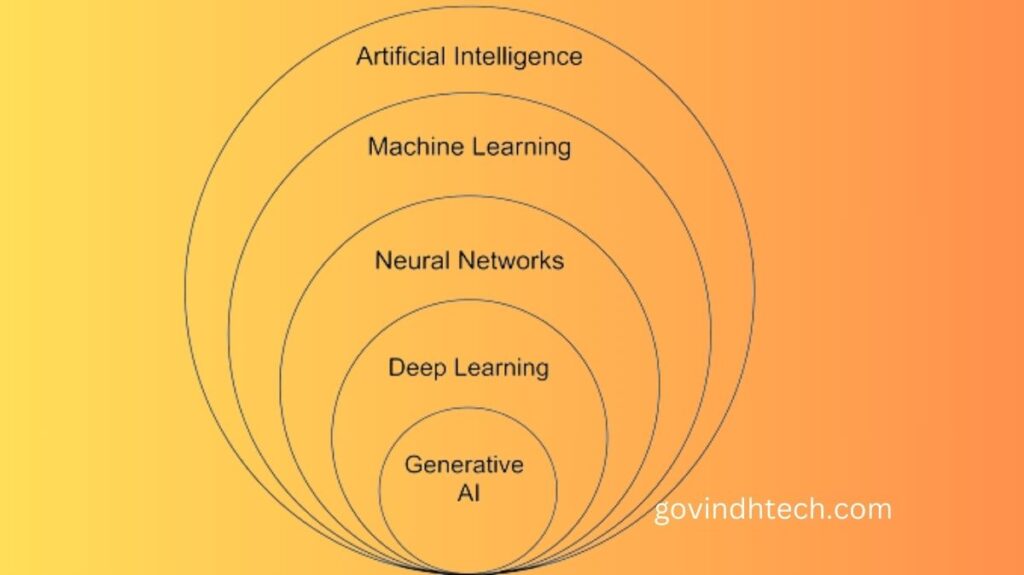
Artificial intelligence involves using models or algorithms to solve an issue like intelligent creatures. These models and algorithms are linked into software programs to make their insights available to users. Some AI solutions use machine learning. Machine learning is an AI area that builds logical or mathematical models that can draw broad inferences from data.
Neural nets, which mimic brain neurons’ networked information flow, are also used in certain AIs. Some advanced neural networks can deep learn and create literature, art, and video. Since deep learning neural nets can now construct these works, they employ the subject category Generative AI.
After sketching the overview, let’s discuss each subfield.
Machine Learning:
Recent years have seen the rise of machine learning, neural networks, and deep learning due to their performance improvements over prior AI types. While another AI may be able to do the same job, each has its own strengths and specialization.
A strength is learning, typically in reaction to data trends. Even a sophisticated AI may not be able to change its model. One of the earliest and most renowned AI instances was the IBM Deep Blue mainframe that defeated Gary Kasparov in the late 1990s. Deep Blue did not learn from its performance versus Gary Kasparov. An experienced team adjusted the supercomputer’s approach between games.
To help machine learning AI training, humans must sanitize, classify, and structure data. Models that require supervision learn supervised. Some models are meant to learn unsupervised and report trends in a dataset without human interaction or data labeling. Semi-supervised learning combines the two.
Neural Networks:
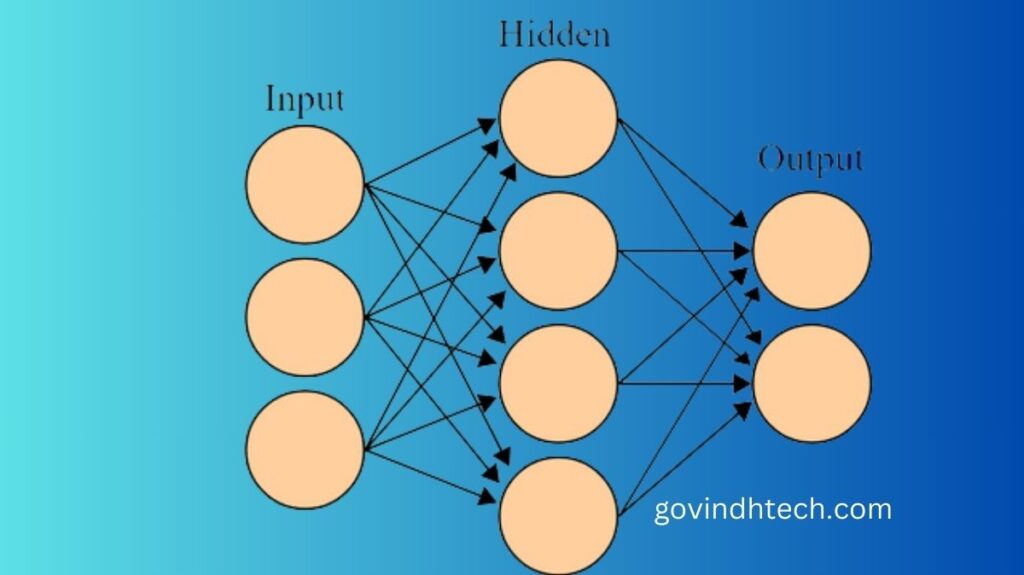
Artificial neural networks are one way to apply machine learning, exactly like AI. Neural nets are computational structures that mimic brain neurons, usually in the human brain and nervous system. Software, hardware, or both may implement neural networks. Brain mimicry why? Brains can do many varied jobs simultaneously in a power envelope smaller than a notepad.
Neural networks have inputs, a hidden layer that processes information, and an output layer. Network nodes are usually called neurons. This example assumes the neural net is feed forward, sending input exclusively to the output layer. More advanced neural networks may backpropagate information to previous levels.
Deep learning:
Deep learning is possible with neural nets with several hidden layers, which may provide higher-level output.
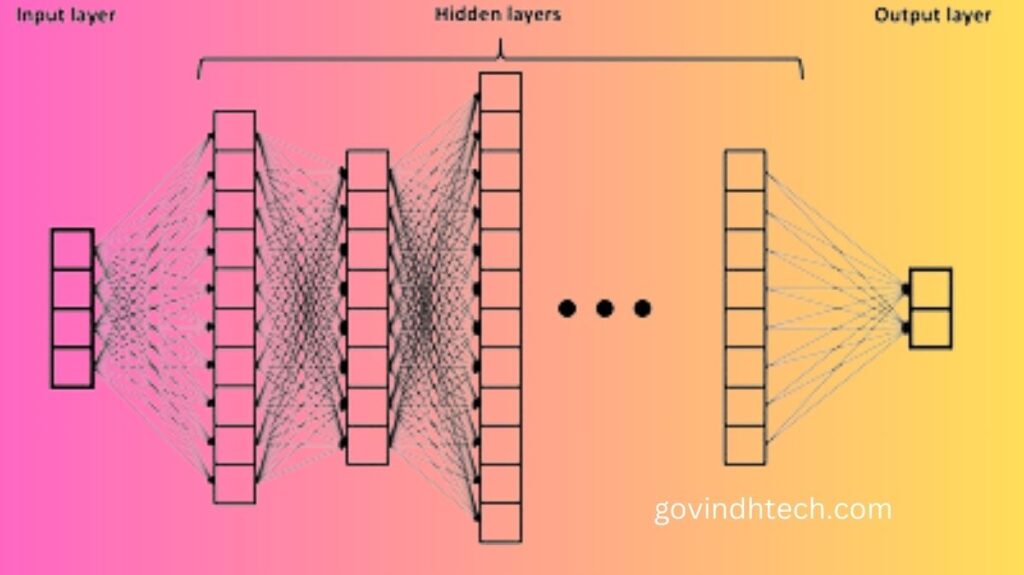
A deep learning neural net is shown in the diagram below. From the left input layer to the right output layer, a single neuron, this neural net processes data. diverse input, hidden, and output layer configurations provide diverse network topologies.
Deep neural nets train models quickly using bigger data sets and high-end technology. The AI model can do more complex tasks including training itself on unparsed and structured data sources by adding layers. Deep learning models provide more complex outputs than basic machine learning approaches but take longer to train.
Generative AI
One of the most promising uses of deep learning is generative AI. A generative AI model may accept user input as a prompt and produce new output in a different format, style, or tone.
Active generative AI models include ChatGPT, GPT-4, Llama 2, and Prometheus (Microsoft). Very big deep learning models that leverage vast datasets can analyze natural language input and training data, powering many generative AIs. These new goods and services aim to develop more proficient bots and helpers.
Generative AI isn’t only a tech trend because firms want the next big thing. Core concepts of neural networks and deep learning were developed long before AI’s popularity. Data storage costs, computation efficiency, and raw performance have improved enough to make deep learning and generative AI commercially viable in the previous decade.
AMD leads this emerging industry with high-end Radeon RX 7000 GPUs and integrated, on-die AI acceleration engines in Ryzen Mobile 7040, 8040, and 8000 desktop CPUs. A larger Ryzen AI initiative aims to support one of the most intriguing technological debuts of the past 40 years by integrating artificial intelligence processing on-die, supporting it with CPU instructions, and building graphics processors that excel in these workloads.
While each generative AI service or hardware company has different ambitions, they did claim that the computer industry’s interest in the issue is motivated by enthusiasm. Science-fiction writers have imagined computers and androids that comprehend and react to humans better than their predecessors in the 20th and 21st centuries. Deep learning and generative AI may not reconcile science fiction and scientific reality, but they are a huge advance over earlier AI methods. Both may be crucial to building artificial intelligences that solve issues like humans rather than computers.