A digital twin is the identical copy of a physical item that exists only in the digital realm. It does this by combining data from the real environment (both in real time and from the past) with engineering, simulation, or machine learning (ML) models in order to improve operations and assist with human decision-making.
Conquer obstacles to maximize the benefits of the digital twin
It is necessary to have a data and logic integration layer in addition to a role-based presentation in order to reap the benefits of having a digital twin. In any asset-intensive business, such as the energy and utility industries, you are required to integrate a variety of data types, as shown in Figure 1. These data sets include things like:
- OT (real-time data from equipment, sensors, and the internet of things)
- Information technology solutions such as enterprise asset management (like Maximo or SAP, for instance)
- Management of the plant’s lifecycle systems
- ERP in addition to a variety of other unstructured data types, including P&ID, visual pictures, and auditory data
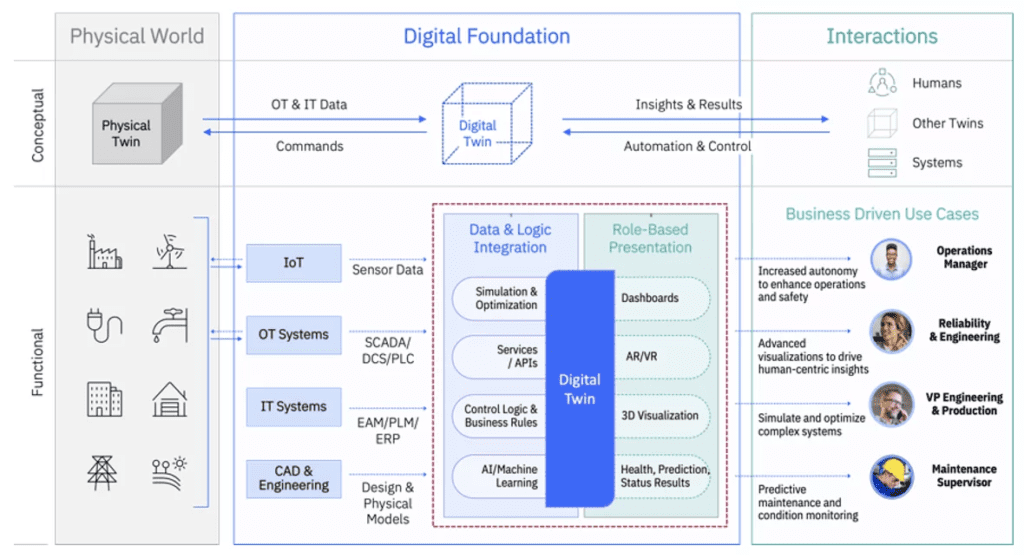
Figure 1. Digital twins and integrated data
For the presentation layer, you can leverage various capabilities, such as 3D modeling, augmented reality and various predictive model-based health scores and criticality indices. At IBM, we strongly believe that open technologies are the required foundation of the digital twin.
When leveraging traditional ML and AI modeling technologies, you must carry out focused training for siloed AI models, which requires a lot of human supervised training. This has been a major hurdle in leveraging data—historical, current and predictive—that is generated and maintained in the siloed process and technology.
As illustrated in Figure 2, the use of generative AI increases the power of the digital twin by simulating any number of physically possible and simultaneously reasonable object states and feeding them into the networks of the digital twin.
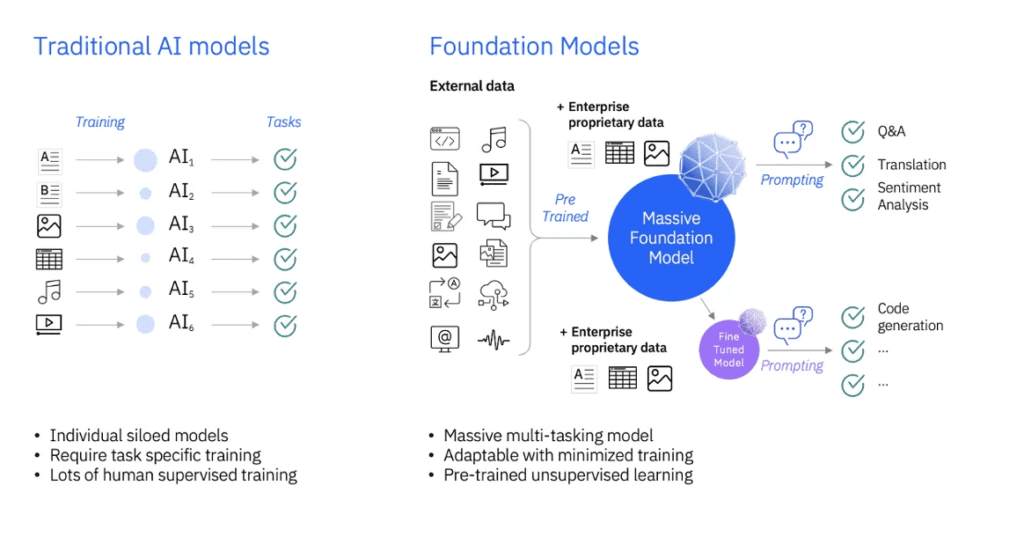
Figure 2. Traditional AI models versus foundation models
The current status of the physical item may be continually determined with the use of these skills. For instance, heat maps may be used to highlight where potential bottlenecks in the power network may arise as a result of an anticipated heat wave that is induced by intensive use of air conditioning (and how these bottlenecks could be alleviated by intelligent switching). In addition to the requirement for an open technical basis, it is essential that the models be reliable and geared toward the business domain.
Use applications for generative artificial intelligence and digital twins in asset-intensive sectors
When generative AI is leveraged for digital twin technologies in an asset-intensive business, such as energy and utilities, several use cases become a reality. Take a look at some of the following use cases that were submitted by our customers in the industry:
1.A look behind the scenes: We are able to make use of the neural network architectures by first developing a fundamental model of a number of different utility asset classes, such as towers, transformers, and lines; then, by using large scale visual pictures; and last, by adapting to the client configuration. We may make use of this to scale the application of artificial intelligence in the identification of abnormalities and damages on utility assets as an alternative to manually evaluating the image.
2.Management of the performance of assets: We build large-scale fundamental models for anomaly detection based on time series data and its co-relationship with other types of data, such as work orders, event prediction, health ratings, criticality index, user manuals, and other types of unstructured data. We make use of the models to construct individual twins of assets, and these twins contain all of the historical knowledge that is relevant to the functioning of the asset in the present and the future.
3.Services in the field: We construct a question-answer feature or multi-lingual conversational chatbot (based on documents or dynamic material from a large knowledge base) that gives field service support in real time by utilizing retrieval-augmented generation activities to create the feature. This technology has the potential to have a significant influence on the performance of field services crews as well as an increase in the dependability of energy services. It does this by providing answers to asset-specific inquiries in real time, without the need to send the end user to documentation, links, or a human operator.
The area of artificial intelligence (AI) faces new challenges as a result of the development of generative AI and large language models (LLMs), and we do not pretend to have all the answers to the concerns that these new solutions raise. IBM is aware that fostering trust and openness in artificial intelligence is not a technological barrier, but rather a socio-technology challenge. This is something that the company is well aware of.
We see a high percentage of artificial intelligence initiatives become stalled in the proof of concept phase for a variety of reasons, including misalignment with business strategy and lack of faith in the model’s outcomes. IBM combines a large amount of experience in the field of transformation with industry expertise as well as proprietary and partner technology. IBM ConsultingTM is in a position to assist organizations in developing the strategy and capabilities necessary to operationalize and scale trusted AI in order to achieve their goals because of the mix of expertise it possesses and the partnerships it maintains.
At the moment, IBM is one of the very few companies on the market that not only offers solutions based on artificial intelligence (AI), but also has a consulting practice that is dedicated to assisting customers in the ethical and responsible application of AI. The Center of Excellence for Generative AI at IBM helps clients build ethically acceptable generative AI solutions and operationalize the whole AI lifecycle.
The process of using generative AI should, among other things: a) be driven by open technologies; b) ensuring that AI is accountable and managed in order to establish confidence in the model; and c) empower those who use your platform. As energy and utility companies work to upgrade their digital infrastructure in preparation for the transition to renewable energy, we think that generative artificial intelligence has the potential to help turn the digital twin promise into a reality. You can become an AI value generator by partnering with IBM Consulting, which gives you the ability to train, deploy, and administer data and AI models on your own.
[…] years will be artificial intelligence models, especially LLMs. Aside from managing and governing AI models, it is crucial to manage and oversee the data fed into the AI since new AI legislation […]
[…] a wider choice of ways to connect with and profit from their company data. In addition to these new gen AI capabilities, we are also executing on this […]
[…] material to address queries from customers and staff. Conversational search employs generative AI to relieve human authors from manually composing and updating replies; this reduces the total cost […]
[…] III international banking regulation requirements, which involve hundreds of pages of paperwork. Gen AI might describe a Basel III region to help a developer comprehend the context, highlight framework […]