We’re all familiar with NVIDIA and the AI “gold mine” that has just taken the world by storm. In the center of it all are Team Green’s H100 AI GPUs, which are the most sought-after piece of AI hardware at the moment, with everyone attempting to get their hands on one to fuel their AI demands.
The NVIDIA H100 GPU is the best chip for AI right now, and everyone wants more of them.
This post isn’t really newsworthy, but it does inform readers on the current state of the AI market and how businesses are revolving around the H100 GPUs for their “future.”
Once we get into the meat of the article, a little summary is in order. So, at the beginning of 2022, everything was proceeding as usual. However, with the entrance of November, a breakthrough application known as “ChatGPT” surfaced, laying the groundwork for the AI frenzy. While “ChatGPT” cannot be considered the originator of the AI boom, it can be considered a catalyst. As a result, competitors such as Microsoft and Google have been forced into an AI race to produce generative AI products.
Where does NVIDIA fit into this, you might ask? The backbone of generative AI is extensive LLM (Large Language Model) training, and NVIDIA AI GPUs come in handy here. We won’t delve into technical details or factual information because it’s boring and uninteresting to read. If you’re looking for specifics, we’ve included a table below that lists every AI GPU release from NVIDIA, dating back to Tesla models.
NVIDIA HPC / AI GPUs
NVIDIA TESLA GRAPHICS CARD | NVIDIA H100 (SMX5) | NVIDIA H100 (PCIE) | NVIDIA A100 (SXM4) | NVIDIA A100 (PCIE4) | TESLA V100S (PCIE) | TESLA V100 (SXM2) | TESLA P100 (SXM2) | TESLA P100 (PCI-EXPRESS) | TESLA M40 (PCI-EXPRESS) | TESLA K40 (PCI-EXPRESS) |
---|---|---|---|---|---|---|---|---|---|---|
GPU | GH100 (Hopper) | GH100 (Hopper) | GA100 (Ampere) | GA100 (Ampere) | GV100 (Volta) | GV100 (Volta) | GP100 (Pascal) | GP100 (Pascal) | GM200 (Maxwell) | GK110 (Kepler) |
Process Node | 4nm | 4nm | 7nm | 7nm | 12nm | 12nm | 16nm | 16nm | 28nm | 28nm |
Transistors | 80 Billion | 80 Billion | 54.2 Billion | 54.2 Billion | 21.1 Billion | 21.1 Billion | 15.3 Billion | 15.3 Billion | 8 Billion | 7.1 Billion |
GPU Die Size | 814mm2 | 814mm2 | 826mm2 | 826mm2 | 815mm2 | 815mm2 | 610 mm2 | 610 mm2 | 601 mm2 | 551 mm2 |
SMs | 132 | 114 | 108 | 108 | 80 | 80 | 56 | 56 | 24 | 15 |
TPCs | 66 | 57 | 54 | 54 | 40 | 40 | 28 | 28 | 24 | 15 |
FP32 CUDA Cores Per SM | 128 | 128 | 64 | 64 | 64 | 64 | 64 | 64 | 128 | 192 |
FP64 CUDA Cores / SM | 128 | 128 | 32 | 32 | 32 | 32 | 32 | 32 | 4 | 64 |
FP32 CUDA Cores | 16896 | 14592 | 6912 | 6912 | 5120 | 5120 | 3584 | 3584 | 3072 | 2880 |
FP64 CUDA Cores | 16896 | 14592 | 3456 | 3456 | 2560 | 2560 | 1792 | 1792 | 96 | 960 |
Tensor Cores | 528 | 456 | 432 | 432 | 640 | 640 | N/A | N/A | N/A | N/A |
Texture Units | 528 | 456 | 432 | 432 | 320 | 320 | 224 | 224 | 192 | 240 |
Boost Clock | TBD | TBD | 1410 MHz | 1410 MHz | 1601 MHz | 1530 MHz | 1480 MHz | 1329MHz | 1114 MHz | 875 MHz |
TOPs (DNN/AI) | 3958 TOPs | 3200 TOPs | 1248 TOPs 2496 TOPs with Sparsity | 1248 TOPs 2496 TOPs with Sparsity | 130 TOPs | 125 TOPs | N/A | N/A | N/A | N/A |
FP16 Compute | 1979 TFLOPs | 1600 TFLOPs | 312 TFLOPs 624 TFLOPs with Sparsity | 312 TFLOPs 624 TFLOPs with Sparsity | 32.8 TFLOPs | 30.4 TFLOPs | 21.2 TFLOPs | 18.7 TFLOPs | N/A | N/A |
FP32 Compute | 67 TFLOPs | 800 TFLOPs | 156 TFLOPs (19.5 TFLOPs standard) | 156 TFLOPs (19.5 TFLOPs standard) | 16.4 TFLOPs | 15.7 TFLOPs | 10.6 TFLOPs | 10.0 TFLOPs | 6.8 TFLOPs | 5.04 TFLOPs |
FP64 Compute | 34 TFLOPs | 48 TFLOPs | 19.5 TFLOPs (9.7 TFLOPs standard) | 19.5 TFLOPs (9.7 TFLOPs standard) | 8.2 TFLOPs | 7.80 TFLOPs | 5.30 TFLOPs | 4.7 TFLOPs | 0.2 TFLOPs | 1.68 TFLOPs |
Memory Interface | 5120-bit HBM3 | 5120-bit HBM2e | 6144-bit HBM2e | 6144-bit HBM2e | 4096-bit HBM2 | 4096-bit HBM2 | 4096-bit HBM2 | 4096-bit HBM2 | 384-bit GDDR5 | 384-bit GDDR5 |
Memory Size | Up To 80 GB HBM3 @ 3.0 Gbps | Up To 80 GB HBM2e @ 2.0 Gbps | Up To 40 GB HBM2 @ 1.6 TB/s Up To 80 GB HBM2 @ 1.6 TB/s | Up To 40 GB HBM2 @ 1.6 TB/s Up To 80 GB HBM2 @ 2.0 TB/s | 16 GB HBM2 @ 1134 GB/s | 16 GB HBM2 @ 900 GB/s | 16 GB HBM2 @ 732 GB/s | 16 GB HBM2 @ 732 GB/s 12 GB HBM2 @ 549 GB/s | 24 GB GDDR5 @ 288 GB/s | 12 GB GDDR5 @ 288 GB/s |
L2 Cache Size | 51200 KB | 51200 KB | 40960 KB | 40960 KB | 6144 KB | 6144 KB | 4096 KB | 4096 KB | 3072 KB | 1536 KB |
TDP | 700W | 350W | 400W | 250W | 250W | 300W | 300W | 250W | 250W | 235W |
The subject of why the H100s remains unanswered. So, we’re getting there. The NVIDIA H100 is the company’s highest-end product, with massive computing capabilities. One could argue that the increase in performance results in higher costs, but firms tend to order in large quantities, and “performance per watt” is the goal here. The Hopper “H100” outperforms the A100 in 16-bit inference and training performance by 3.5 times, making it the obvious choice.
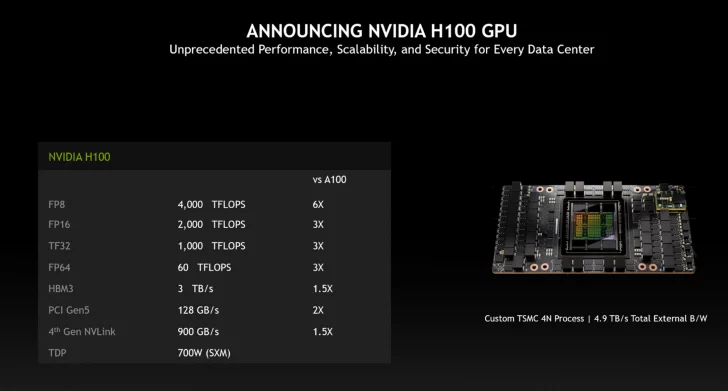
Therefore, we’re hoping that the superiority of the H100 GPU is clear here. Moving on to our next topic, why is there a scarcity? The answer incorporates multiple factors, the first of which is the massive number of H100s required to train a single model. Astonishingly, OpenAI’s GPT-4 AI model required 10,000 to 25,000 A100 GPUs (H100s were not available at the time).
Advanced artificial intelligence businesses such as Inflection AI and CoreWeave have acquired massive amounts to H100s, totaling billions of dollars. This demonstrates that even to train a basic-to-decent AI model, a single organization requires massive volumes, resulting in massive demand.
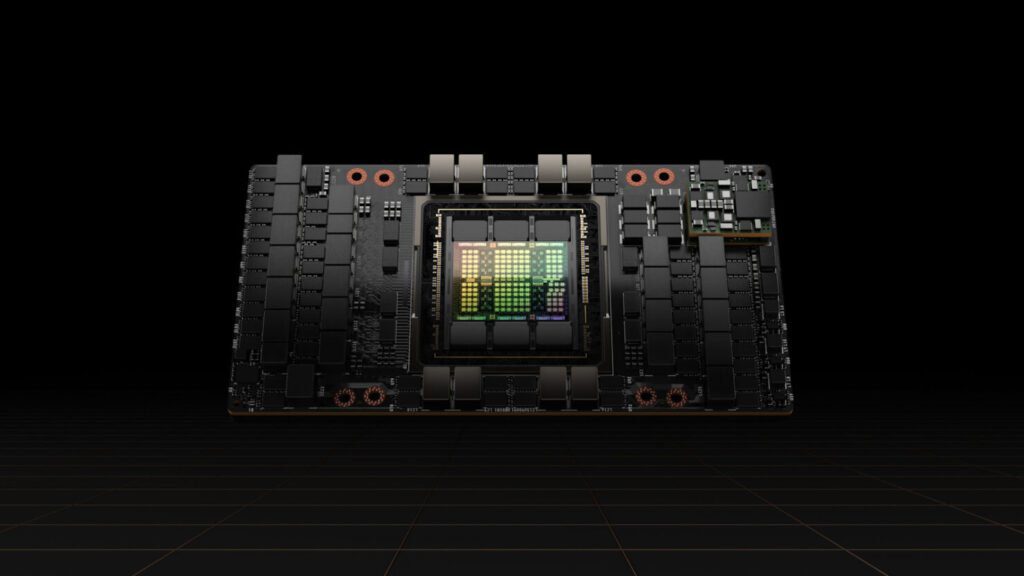
If NVIDIA’s method is questioned, one can respond, “NVIDIA could increase production to meet demand.” It is much easier to say this than it is to do it. Unlike gaming GPUs, NVIDIA AI GPUs necessitate sophisticated processes, with the majority of production delegated to Taiwanese semiconductor titan TSMC. TSMC is NVIDIA’s sole supplier of the AI GPU, overseeing all processes from wafer purchase through sophisticated packaging.
The H100 GPUs are built on TSMC’s 4N technology, which is a redesigned variant of the 5nm series. NVIDIA is the most important customer for this method because Apple previously used it for its A15 Bionic chipset, which has since been superseded by the A16 Bionic. The creation of HBM memory is the most difficult of all critical phases since it requires advanced equipment that is only used by a few manufacturers.
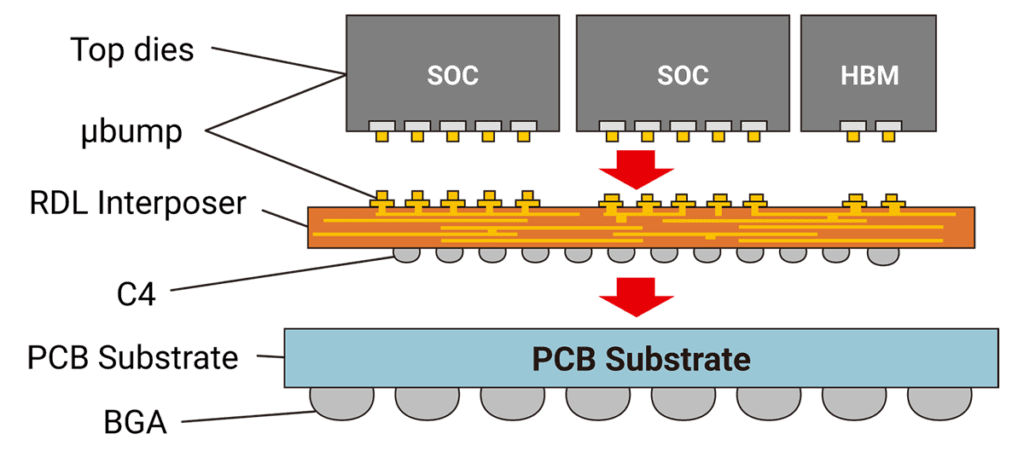
HBM vendors include SK Hynix, Micron, and Samsung, although TSMC has limited its suppliers, the identities of whom we are unclear. Aside from HBM, TSMC is also struggling to maintain CoWoS (Chip-on-Wafer-on-Substrate) capacity, a 2.5D packaging technique, and a critical stage in the development of H100s. Because TSMC is unable to meet NVIDIA’s demand, order backlogs have reached record heights, with delivery delayed until December.
We have left out numerous facts because going into detail would detract from our core goal of informing the ordinary user about the situation. While we do not believe the scarcity will be alleviated very soon, it is expected to worsen. However, with AMD’s decision to consolidate its position in the AI sector, we may witness a shift in the landscape.
According to DigiTimes, “TSMC appears to be particularly optimistic about demand for AMD’s upcoming Instinct MI300 , claiming that it will account for half of Nvidia’s total output of CoWoS-packaged chips.”” It is possible that the burden will be distributed across organizations. Still, given Team Green’s greedy policies in the past, something like this would necessitate a significant offering from AMD.
To summarize our discussion, NVIDIA’s H100 GPUs are driving the AI craze to new heights, which is why they are surrounded by such a frenzy. We hoped to conclude our discussion by providing readers with a general overview of the situation. GPU Utilis deserves credit for the inspiration for this article; be sure to read their report as well.
[…] NVIDIA H100 Tensor Core GPUs and NVIDIA Quantum-2 InfiniBand networking used in the Microsoft Azure ND H100 v5 VMs, which are already […]
[…] NVIDIA GH200 Grace Hopper platform, developed for the age of accelerated computing and generative AI, is now available. It is based on a new Grace Hopper Superchip and has the first HBM3e processor in […]
[…] and a single desktop workstation may deliver up to 5,828 TFLOPS of AI capability and 192GB of GPU memory. Systems can be configured with NVIDIA AI Enterprise or Omniverse Enterprise, depending on […]
[…] to the Cost of a Data Breach 2023 worldwide survey, employing artificial intelligence (AI) and automation greatly helped enterprises by saving them up to USD 1.8 million in expenses […]
[…] edge over those that do not fully integrate AI into their processes if they are the first to adopt AI and use it ethically and efficiently to grow revenue and enhance operations. Here are some crucial […]
[…] organization comes with dangers and difficulties. These include restrictions on the development of AI, security and data leakage, confidentiality and liability issues, intellectual property challenges, […]
[…] everyone has the financial means to rent or purchase an Nvidia H100 (Hopper) to conduct AI experiments. Even if you have the money, you might not be able to get a […]
[…] traditional ML and AI modeling technologies, you must carry out focused training for siloed AI models, which requires a lot of human supervised training. This has been a major hurdle in […]
[…] company promotes the Emulation Protection software that it has developed as a “revolutionary technology in the fight against […]
[…] fast-forward to a classroom with plenty of technology before, during, and after COVID. How has technology altered this […]
[…] for the Age of Artificial Intelligence, Samsung Electronics Has Introduced the Industry’s Highest-Capacity 12nm-Class 32Gb DDR5 […]
[…] The researchers have created a novel technique for identifying and monitoring coral reef halos, which are rings of bare sand around reefs, using deep learning models and high-resolution satellite images powered by NVIDIA GPUs. […]
[…] Compute bare-metal instances with NVIDIA H100 GPUs, which are powered by the NVIDIA Hopper architecture, provide an order-of-magnitude jump for […]
[…] that access to high-end H100s was previously restricted, the US regulation shift is likely to impede the expansion of AI markets […]
[…] V100 is a PCIe Gen3 GPU, and NVIDIA’s GPU generations are advancing at a rate that is far faster than PCIe and platform generations. They […]
[…] expressions, may be placed anywhere in a room. In the context of the dialogue, it reacts. Romi is an AI-powered robot that goes beyond what AI has been utilized for up until this point to do, which is to […]