The AI Infrastructure aims to unpack the different facets and their respective computational requirements, showing that AI investments must be based on long-term business outcomes and values.
Optimized AI Infrastructure
In recent years, AI technology providers have progressively reduced the barriers to entry by actively launching innovative products and services. Graphic Processing Units (GPUs) and Application-Specific Integrated Circuits (ASICs) are being adopted for AI training and inference.Nowadays, more and more general-purpose Central Processing Units (CPUs) can support AI inference and training. The introduction of pre-training language models allows developers to build complex applications, such as speech recognition and machine translation, without training a model from scratch. Auto Machine Learning (AutoML) provides methods, tools, and techniques to make the development process easier for non-AI experts by automating AI workflow.
KEY PRINCIPLES OF AI INFRASTRUCTURE INVESTMENT
Today’s AI is narrowly focused, requires a wide range of expertise, and exists in a silo. In contrast, the AI of tomorrow requires enormous amounts of resources and deep technological knowledge,which remains out of reach for most businesses. Therefore, businesses must start early, identify the business outcomes that cannot be easily achieved without AI, actively build internal capabilities, and roll out these advanced AI techniques widely across the entire organization.
AI Infrastructure Must Be Driven by Business Outcomes
Understanding the intended business outcomes of AI deployment is crucial. It ensures that AI projects align with the organization’s goals and have tangible financial value recognized by senior management.
Heterogeneous and Flexible AI Infrastructure
- Scaling up and scaling out AI applications is important for maximizing benefits. AI infrastructure should be designed to support different facets of AI model design, development, and deployment across various computing platforms.
- A heterogeneous compute platform, utilizing different types of hardware (e.g., CPU, GPU, ASIC), allows for optimized performance across different AI tasks, such as data gathering, model training, and inference workloads.
Backward Compatibility of AI Infrastructure
AI infrastructure should be compatible with existing enterprise solutions to avoid creating silos in the business operation. Ensuring versatility, robustness, and interoperability is essential for optimizing IT/OT infrastructure and processes.
Open and Secure AI Infrastructure
- Openness in AI infrastructure, both in terms of hardware and software, promotes interoperability with other solutions and prevents vendor lock-in.
- While being open, AI infrastructure must prioritize state-of-the-art cybersecurity and data protection mechanisms to safeguard against hacking, protect user data, and comply with legal requirements.
CHARACTERISTICS OF A FUTURE-PROOFED AI
INFRASTRUCTURE
Businesses must understand that building AI for business is a continuous process involving many building blocks. As shown in Figure several key recent advancements have allowed AI to become a reality.

COMPREHENSIVE AND HETEROGENOUS INFRASTRUCTURE
AI inference and training workloads rely more and more on parallel accelerated computing capabilities. The explosive demand for GPUs and AI accelerators is a clear sign of the critical role of accelerated computing in the age of AI. However, AI is more than just peak computing performance or a high degree of optimization for specific applications. AI hardware with a high degree of specialization is excellent in handling specific AI models, but it could be overwhelmed when handling AI models not optimized for the specific hardware.
Computing: Most AI training and inference today are based on GPU, thanks to their parallel processing ability and graph processing DNA, which is considered a great asset for processing AI workloads. Not surprisingly, cloud AI giants and enterprises are expected to continue investing in GPU-optimized systems for AI training and inference workloads in the cloud. In recent years, the emergence of high-performing and power-efficient ASICs has played a crucial role in further accelerating AI inference workloads due to their massive parallel computing performance and credit to their ability to process some complex functions in the hardware domain.
Storage: Data are the most critical assets in AI training. To achieve high performance, the storage system for AI infrastructure needs to be smart at managing data flow, priority, and access. Key attributes include high read and re-read performance, good write I/O, multitier storage hierarchy, public cloud access, multi-protocol support, security, and extensible metadata to facilitate data classification. For businesses dealing with ultra-large datasets, purpose-built architecture is more cost-effective.
Networking: As AI requires large amounts of data for training and testing, the system needs a network topology that can handle a high volume of both north/south (server to storage) traffic and east/west (server to server) traffic. Dedicated chipsets have emerged in recent years capable of orchestrating data movement from different storage tiers, moving data from the edge to the primary storage and data lake, then into the data prep staging tier, and finally into the training cluster.
Software: The final component of sound AI infrastructure is an AI development platform that supports major AI frameworks and all the reference models, kernel libraries, containers,firmware, drivers, and tools. The platform must perform infrastructure provisioning and management, orchestration, and job scheduling during AI training and inference. Once AI is tested and validated, it must be deployed in the field. AI workflow management tools help ensure data management and monitoring, bias and model drift, model retraining, and upgrade. In other words, developers should not need to manage their AI workloads manually. Instead, the software should orchestrate the entire process, enabling developers to do what they are best at developing innovative AI models that address key business pain points.
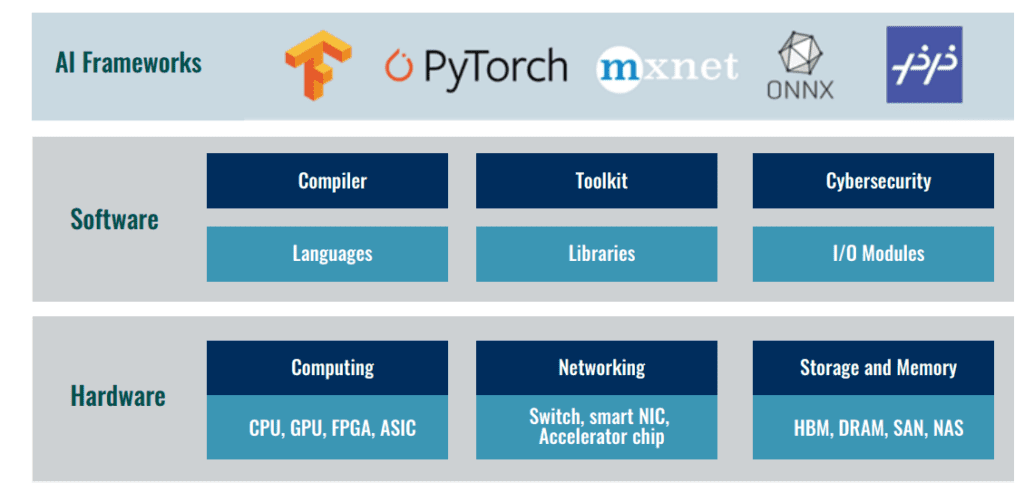
EDGE-TO-CLOUD VISION
An edge-to-cloud strategy should include processing data, and training, deploying, monitoring, and maintaining models at every node of the distributed computing architecture. Understanding the nature of each AI application allows businesses to deploy AI models at the most optimal compute node.
AI Infrastructure Must Be Backward Compatible: All AI infrastructure must be able to work with existing enterprise solutions. Therefore, setting a versatile, robust, and interoperable foundation with all existing solutions is a must. Incompatibility risks creating many silos in the business operation, leading to poorly optimized IT/OT infrastructure and processes.
AI Infrastructure Must Be Open and Secure: Businesses always want to avoid vendor locking. An AI infrastructure consisting of open hardware and software that can interoperate with other solutions is significant in ensuring smooth IT/OT processes. At the same time, openness should not lead to a compromise in security. The AI foundation must feature state-of-the-art cybersecurity and data protection mechanisms to prevent hacking, protect user data, and comply with legal requirements.
[…] and Head of DRAM Marketing at SK hynix. “Along with a faster advancement in the broader IT industry.” “The business will maintain its dominance in the premium memory market by […]
[…] best-of-breed strategy for GenAI products. Customers of Dell now have even more choices for managing IT resources and GenAI infrastructure. VMware and NVIDIA aim to democratize GenAI and spark business innovation […]
[…] important for companies that want to obtain a competitive advantage in their industry. Building an AI infrastructure from the ground up, however, may be a challenging endeavor, particularly for those who are just […]