KASIKORN Business-Technology Group (KBTG) Labs, a prominent tech company in Thailand, has partnered with Muang Thai Insurance PCL (MTI) to leverage machine learning (ML) technology in order to expedite the process of assessing vehicle damage and evaluating insurance claims. The current manual process involves repair shops assessing the damage and submitting claims to the insurance company, which are then validated and processed with the involvement of multiple partners and intermediaries.
To address the challenges posed by this manual process, KBTG Labs collaborated with Google Cloud to devise a solution architecture. The existing dataset used for claims processing had issues related to data quality and quantity, such as incorrect descriptions and images taken from inappropriate distances. Google Cloud proposed a solution architecture that incorporates a cascaded model approach, including image quality assessment.
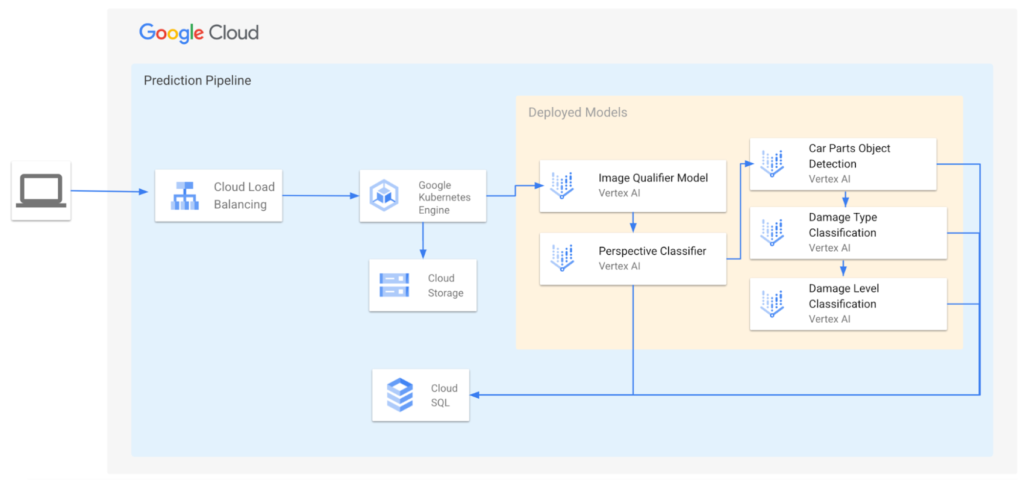
The proposed architecture consists of an inference pipeline designed for scalability, high availability, and fault tolerance. A microservice, served by Google Kubernetes Engine, handles model execution and selection based on inputs and outputs of intermediary models. The images are stored in Cloud Storage and sent for prediction in a bucket, to be labeled for continuous training. Cloud SQL stores the inference results for analytics. The Image Qualifier model, utilizing Vertex AI, classifies input images as good or bad, acting as a filter for image quality and ensuring that only images conforming to quality standards are processed further.
The team conducted experiments to distinguish between “good” and “bad” images using the Image Qualifier model. They found that distance played a significant role in image quality, and using images with appropriate distance metrics improved the Precision and Recall of the ML model by approximately 30%. The experiments revealed that different distance labels, such as Distance 1, Distance 2, and Distance 3, had varying impacts on the model’s performance.
To enhance the interpretability of the image classification, the team employed Explainable AI, a feature of Vertex AI. Explainable AI generates feature attributions or importance values that illustrate how much each input feature contributes to the model’s predictions and overall predictive power. By using Explainable AI, the team was able to gain insights into the model’s behavior and optimize its performance. For instance, the team could identify areas where the model focused on car lights for break defect identification.
Initially, the architecture considered damage classification and object detection separately. However, based on the insights from Explainable AI, it was determined that damage identification should be performed along with object detection to minimize the influence of background objects on the detection process.
Key learnings from the project included the importance of photographing objects from an appropriate distance to facilitate accurate vehicle damage detection and the need to minimize the influence of background objects. As the next step, the team plans to evaluate the results of the experiment, particularly regarding the object detection process, with the aim of further improving the overall system.
[…] A Rapid Brain Tumor Analysis With AI Identify vehicle damage with Machine Learning UNIDO and Huawei Launch AI for Manufacturing Google Gemini AI: The Future of […]
[…] many companies are unable to activate data and AI because their data systems for analytics, AI, and machine learning are fragmented. These data systems weren’t built to function together and instead impede down […]
[…] from the real environment (both in real time and from the past) with engineering, simulation, or machine learning (ML) models in order to improve operations and assist with human […]
[…] also known as text data mining, is an advanced data science subject that employs NLP, AI, and machine learning models to extract qualitative information from unstructured text data. Text analysis focuses on pattern […]
[…] support has transformed our workflow. Our application design combines foundation and proprietary ML models to overcome real-time content personalisation scalability issues using Google Cloud’s […]
[…] workloads, the richness of data in each transaction, and the data in log files are gold mines for machine learning and AI applications to exploit cognitive capabilities and create a more intelligent and secure […]
[…] now feel a greater need to integrate analytics and machine learning into one of their most important technological environments: data, thanks to recent developments in […]
[…] look at machine learning via the prism of AMD Instinct accelerators: The realm of intelligent […]
[…] applications such as artificial intelligence, machine learning, and big data are causing modern businesses to deal with ever-increasing amounts of data flow […]
[…] QRadar Suite, which comprises EDR, log management and observability, SIEM, and SOAR, delivers AI, machine learning (ML), and automation capabilities throughout its integrated threat detection and response […]
[…] Machine learning (ML) can be used in computer vision, large language models (LLMs), speech recognition, self-driving cars, and many more use cases to make decisions in healthcare, human resources, finance, and other areas. […]