Contents
Data Loss Prevention Use Cases
DLP is now a fundamental component of every sector’s cybersecurity strategy for modern businesses. Let’s examine a few of the most important Use Cases.
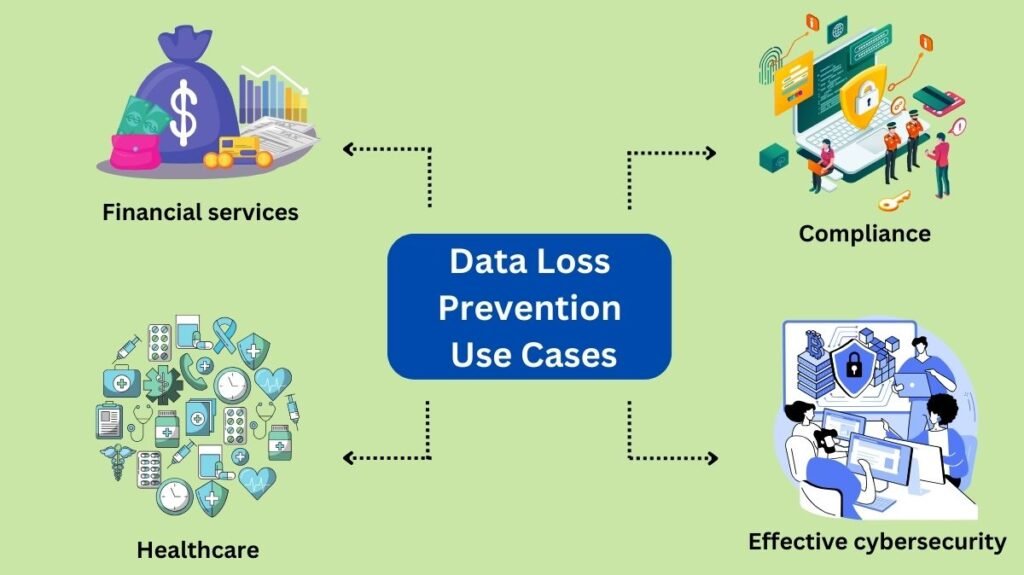
Financial services
Malicious actors frequently target the financial services sector. Financial institutions save credit card numbers, bank account numbers, social security numbers, M&A information, and intellectual property. In other words, banks probably have it if it’s valuable.
Financial organisations must monitor both external and internal threats. Financial services is one of the most competitive industries, making it exposed to insider attacks.
Due to banks’ massive amounts of sensitive data and its risks, the business is highly regulated. Financial institutions must follow GLBA, COPPA, FACTA, HIPAA, CCPA, and GDPR.
A strong DLP tool is essential to overcoming all of these obstacles. DLP can help with compliance auditing, insider threat mitigation, and stopping data exfiltration.
Healthcare
The sensitive nature of the patient data handled by the healthcare sector puts it under constant regulatory and auditing inspection. In this regard, regulators are harsh on errors or carelessness, as evidenced by the high penalties levied for infractions.
Healthcare organisations, like other organisations, suffer with human error in spite of compliance demands. A HIPAA fine might be triggered simply by an employee sharing data with the incorrect person.
Furthermore, because healthcare organisations provide essential services, fraudsters target them explicitly because they are more inclined to comply with demands.
The fact that 80% of healthcare data now travels via the cloud only serves to exacerbate these difficulties. The emergence of cloud-based internal collaboration tools, mobile applications, and teleconsultations enhances patient experience and staff collaboration while simultaneously increasing the danger of data loss.
Applications like Google and Slack don’t have native data security features with the level of detail needed to comply with HIPAA. As a result, using a third-party DLP tool is crucial. DLP offers unmatched visibility and control over data flow, enabling it to identify and safeguard PHI/PII across cloud environments.
Compliance
Using DLP tools is required by several compliance rules, and any audit will verify this.
Naturally, complying with regulations such as the CCPA and GDPR can be a laborious task. However, organisations may automate a number of compliance-related tasks with the correct DLP tool.
First, by locating personal data within the company, even in Software-as-a-Service (SaaS) applications, DLP facilitates its identification. Understanding the locations of sensitive information and making sure it is appropriately maintained in accordance with legal requirements depend on this proactive identification.
Additionally, DLP makes compliance easier by putting policies in place like deleting and encrypting sensitive data when needed. By taking these steps, organisations can better comply with regulations for the safe management of private and sensitive data.
DLP not only protects data but also stops unwanted access to private data. DLP systems reduce the possibility of data breaches and tampering incidents by limiting the danger of unauthorised users accessing or altering data by the enforcement of access rules and real-time monitoring of data access activities.
DLP solutions also enforce compliance guidelines throughout the company. They provide the uniform application and upkeep of data security standards and legal obligations. In addition to bolstering data security protocols, this proactive enforcement assists businesses in avoiding fines and harm to their brand that come with noncompliance.
Effective cybersecurity
Data loss prevention (DLP) solutions are crucial for protecting organisations from intentional hostile activity as well as unintentional data disclosure, even beyond compliance requirements.
By limiting access to sensitive data to authorised workers, these solutions improve security at the data-access level. DLP solutions stop unauthorised people from accessing private information by implementing stringent access controls and keeping an eye on data usage in real time. This feature greatly reduces the possibility of data breaches either from compromised credentials or internal sources.
Furthermore, DLP systems go one step further by guaranteeing that authorised users manage private data sensibly and in compliance with corporate and legal guidelines. They keep an eye on and limit the usage of data, avoiding abuse or unapproved distribution that can result in infractions of the law or harm to one’s reputation.
Advantages of Preventing Data Loss
Enhanced Data Security
DLP solutions monitor and regulate the sharing and access of data, offering strong security against data breaches. By implementing stringent security regulations, they assist avoid unintentional or deliberate data breaches by guaranteeing that private information stays within permitted bounds.
Regulatory Compliance
Maintaining compliance with evolving rules like the CCPA, GDPR, and HIPAA is essential. By finding and preserving regulated data, DLP systems help firms avoid steep fines and legal action.
Prevention of Insider Threats
Insider attacks are among the most disregarded security threats. Employee behaviour is monitored by DLP systems to identify anomalous actions that can jeopardise data security, such downloading or sharing private files without authorisation.
Intellectual Property Protection
Cutting-edge firms need IP protection. DLP helps the organisation stay competitive by protecting trade secrets, patents, and designs.
Business Continuity
Reputations can be damaged and operations disrupted by data breaches. DLP solutions help ensure smooth company continuity by avoiding data loss, which lowers downtime and protects consumer confidence.
A Better View of the Data Flow
Granular insights into the flow of data inside an organisation are provided by DLP solutions. By revealing network vulnerabilities, this openness enhances the security architecture as a whole.
Disadvantages of Data Loss Prevention
High Implementation Costs
DLP system deployment, configuration, and maintenance costs might be too high for small and medium-sized businesses (SMEs). The cost of employing qualified experts to oversee these systems also rises.
Complex Deployment
Because of its complexity, DLP can be difficult to implement. It frequently needs to be heavily customised to meet the unique requirements of the company, which might result in protracted deployment periods.
False Positives
Frequently, DLP systems identify harmless activities as security risks, resulting in “false positives.” By slowing down procedures, this might cause operational delays and irritate staff.
Overhead for Performance
System performance may be slowed down by the ongoing monitoring and analysis carried out by DLP technologies, especially in big organisations with substantial data quantities.
Resistance from Employees
Strict data controls enforced by DLP may encounter pushback from staff members who perceive the system as invasive. To solve such issues, effective communication and training are crucial.
Limited Protection Against Sophisticated Attacks
DLP may not completely defend against extremely sophisticated cyberattacks or zero-day vulnerabilities, despite its effectiveness against several threats. Comprehensive protection frequently necessitates complementary security measures.
Types of Data Loss Prevention
Different DLP techniques are used to safeguard data at various organisational stages and locations:
Data Identification
This entails searching through and cataloguing private data on a company’s network. To guarantee that only authorised individuals may access vital data, it incorporates cloud storage, email, and collaboration tools.
Identification of Data Leak
In order to enable quick repair, DLP uses automation to identify and locate unauthorised data movements or exposures inside the organization’s infrastructure.
Data-in-Motion
DLP uses encryption and other security measures to secure data while it moves between network points or locations, guaranteeing data privacy and integrity.
Data At-Rest
Data kept on physical devices or in cloud settings is covered by this protection. To stop unwanted access or changes to stored data, it incorporates encryption, access restrictions, and other security features.
Utilised Data
DLP makes ensuring that user-accessed or user-manipulated data isn’t abused. Controls on data handling operations, such as copying, editing, printing, and monitoring to identify questionable user behaviour, are examples of protection measures.