Nvidia ConsiStory Consistent Text-to-Image Generation Without Training. Without further training, can allow Stable Diffusion XL (SDXL) to provide consistent subjects throughout a sequence of photos.
It introduce ConsiStory, a training-free method for pretrained text-to-image models that allows for consistent subject creation. Because it doesn’t require customization or fine-tuning, it generates images on an H100 in around 10 seconds, which is 20 times quicker than earlier state-of-the-art techniques. To improve topic consistency across pictures, one can add a correspondence-based feature injection and a subject-driven shared attention block to the model.
Furthermore, experts create plans to promote layout variety while preserving subject coherence. Nvidia ConsiStory can easily expand to multi-subject situations and even allow for ordinary things to be personalized without training.
ConsiStory: AI-Produced Pictures With Vitality for the Main Character
Researchers from NVIDIA and Tel Aviv University collaborated to create ConsiStory, a tool that facilitates the creation of many pictures featuring a single main character. This feature is crucial for storytelling use cases like creating a storyboard or designing a comic strip.
By using a technique known as subject-driven shared attention, the researchers were able to cut down the time required to produce consistent visuals from 13 minutes to about 30 seconds.
How does Nvidia ConsiStory works?
- Architecture outline (left): Everyone localize the subject in each generated image 𝐼𝑖 at each generation stage based on a series of prompts. To construct topic masks 𝑀𝑖, then use the cross-attention maps up to the present generation step. Next, next swap out the U-net decoder’s conventional self-attention layers with Subject Driven Self-Attention layers, which exchange data between subject instances. For further enhancement, could also incorporate Feature Injection.
- Subject Driven Self-Attention: To expand the self-attention layer such that, subject to their subject masks 𝑀𝑗, the Query from produced picture 𝐼𝑖 will also have access to the Keys. In order to increase variety, the use dropout to weaken the SDSA and combine query features from a non-consistent sampling phase with vanilla query features to produce 𝑄.
- Feature Injection (right): Lets implement a method for combining characteristics inside the batch to further hone the subject’s identification across pictures. After extracting a patch correspondence map between every pair of photos, that are utilized that map to inject features between the images.
Nvidia ConsiStory
Comparison To Current Methods
It tested the methodology against TI, DB-LORA, and IP-Adapter. Some approaches did not adhere to the prompt (IP-Adapter) or preserve consistency (TI). Other approaches alternated between following the text and maintaining consistency, but not both (DB-LoRA). This strategy was consistent while effectively adhering to the request.
Quantitative Evaluation
- Automatic Evaluation (left): Nvidia ConsiStory (green) strikes the ideal ratio between textual similarity and subject consistency. While optimization-based techniques like LoRA-DB and TI do not show the same level of topic consistency as this approach, encoder-based techniques like ELITE and IP-Adapter frequently overfit to visual appearance. Different self-attention dropout levels are indicated by 𝑑. S.E.M. error bars are used.
- User Study (right): findings show that users significantly preferred the produced pictures in terms of both Textual Similarity (Textual) and Subject Consistency (Visual).
Multiple Consistent Subjects
Image collections with several constant subjects may be produced with ConsiStory.
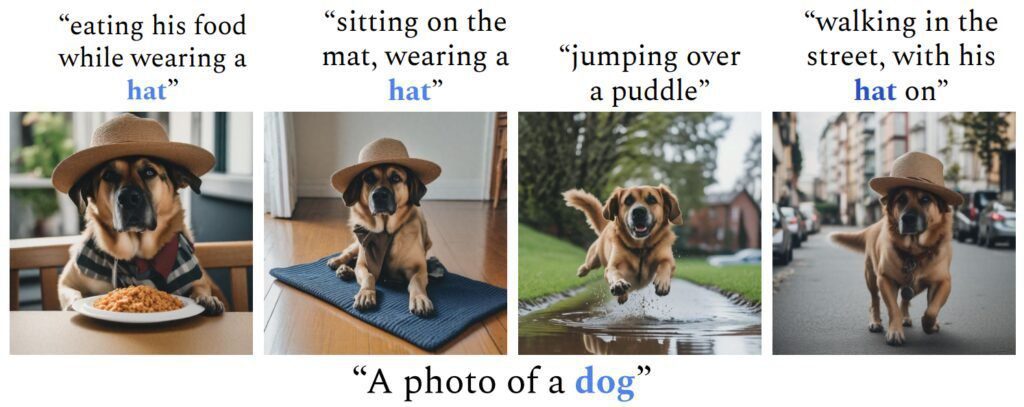
Abstraction
By enabling users to direct the picture generating process using natural language, text-to-image models provide a new degree of creative flexibility. It is still difficult to use these models to depict the same subject consistently across a variety of stimuli. Current methods either add image conditioning to the model or refine it to learn additional words that characterize certain subjects supplied by the user.
These techniques need extensive pre-training or protracted per-subject optimization. They also have trouble displaying several subjects and aligning produced graphics with written suggestions. Here, the introduce Nvidia ConsiStory, a training-free method that shares the pretrained model’s internal activations to enable consistent subject creation. To encourage subject consistency across pictures, researchers provide a correspondence-based feature injection and a subject-driven shared attention block.
In addition, create plans to promote layout variety while preserving subject coherence. Without the need for a single optimization step, everyone show state-of-the-art performance on topic consistency and text alignment when comparing ConsiStory to a variety of baselines.
Last but not least, Nvidia ConsiStory may easily expand to multi-subject situations and even allow for training-free customization of everyday things.