Google DeepMind at NeurIPS 2024
What is NeurIPS?
The Conference on Neural Information Processing Systems, or NeurIPS, is an annual gathering of scholars from the domains of statistics, computational neuroscience, artificial intelligence, and machine learning:
Google DeepMind teams will present over 100 new papers on anything from generative media and AI agents to cutting-edge learning strategies.
Developing Safe, Intelligent, and Adaptive AI Agents
When given natural language instructions to do digital tasks, LLM-based AI agents are demonstrating promise. However, their ability to precisely engage with intricate user interfaces which calls for a large amount of training data is essential to their success. It believes that the substantial performance improvements demonstrated by AI agents trained on this dataset will contribute to the development of more general AI agents.
In order to generalize across tasks, AI agents must learn from every experience they have. Its approach to in-context abstraction learning improves agents’ performance and adaptability by assisting them in understanding important task patterns and correlations from natural language feedback and incomplete demos.
A frame from a video demonstration showing the distinct components of a sauce being numbered and recognized. ICAL can identify the crucial elements of the procedure.
Creating agentic AI that strives to achieve users’ objectives might increase the technology’s utility, but when creating AI that acts on its behalf, alignment is crucial. It demonstrates how a model’s perception of its user might affect its safety filters and offer a theoretical approach to quantifying the goal-directedness of an AI system. When taken as a whole, these observations highlight how crucial it is to have strong protections against unwanted or dangerous behaviour so that AI agents’ actions stay in line with safe, intended applications.
Enhancing 3D simulation and scene creation
The need for high-quality 3D content is increasing in sectors like visual effects and gaming, yet producing realistic 3D scenarios is still expensive and time-consuming. In its latest study, it presents new methods for 3D generation, simulation, and control that simplify content development for quicker, more adaptable workflows.
It is frequently necessary to take and model thousands of 2D images in order to create realistic, high-quality 3D sceneries and assets. Google DeepMind presents CAT3D, a system that requires only a single image or a written prompt and can produce 3D material in as little as one minute. CAT3D achieves this by using a multi-view diffusion model to provide more consistent 2D images from a wide range of perspectives. These images are then used as input for conventional 3D modeling methods. Results outperform earlier techniques in terms of speed and quality. With CAT3D, any number of produced or actual photos can be used to create 3D scenes.
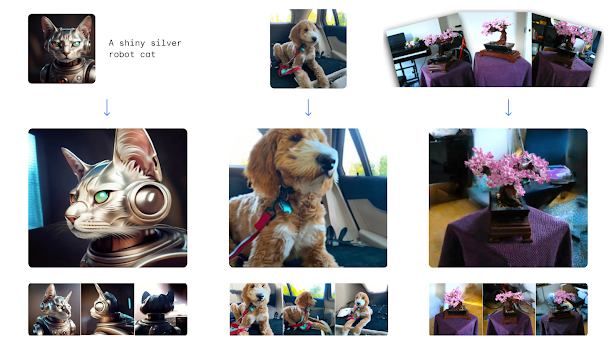
CAT3D enables 3D scene creation from any number of generated or real images.
A real photo to 3D, multiple photos to 3D, and text-to-image-to-3D are shown from left to right.
A cluttered tabletop or falling Lego blocks are examples of scenarios containing a lot of stiff items that are nevertheless computationally demanding to simulate. Google DeepMind introduces SDF-Sim, a novel method that enables effective simulation of big, complex scenes and accelerates collision detection by representing object forms in a scalable manner.
Multiple objects’ 3D location and orientation are difficult for AI picture generators based on diffusion models to govern. Its method, Neural Assets, presents object-specific representations that are learned from dynamic video input and capture both appearance and 3D posture. Users can move, rotate, or switch items between scenes with Neural Assets, which is a helpful feature for virtual reality, animation, and gaming. With a source image and object 3D bounding boxes, it can transfer objects or backgrounds across images or translate, rotate, and rescale the object.

Given a source image and object 3D bounding boxes, we can translate, rotate, and rescale the object, or transfer objects or backgrounds between images
Enhancing LLMs’ learning and reaction
Additionally, by boosting LLMs’ training, learning, and user response, is enhancing efficiency and performance in a number of ways.
Larger context windows have made it possible for LLMs to learn from thousands of instances simultaneously, a technique called many-shot in-context learning (ICL). This procedure improves the model’s performance on tasks like reasoning, translation, and math, but it frequently calls for high-quality, human-generated data. To eliminate the need for manually selected material, it investigates ways to alter many-shot ICL to make training more economical. Since there is so much data accessible for language model training, teams’ primary limitation is the amount of computing power they have. Google answers a crucial query: how do you select the ideal model size to get the best outcomes when you have a limited computing budget?
It refers to this novel method as Time-Reversed Language Models (TRLM), which investigates pretraining and optimizing an LLM to operate in reverse. When input is typical LLM responses, a TRLM creates queries that may have resulted in such responses. When combined with a conventional LLM, this approach not only makes sure responses better adhere to user instructions, but it also increases safety filters against hazardous content and generates better citations for summarized text.
Large-scale manual curation is challenging, yet it is essential for training AI models. By finding the most learnable data in larger batches, our Joint Example Selection (JEST) algorithm optimises training and outperforms state-of-the-art multimodal pretraining baselines, allowing for up to 13× fewer training cycles and 10× less processing.
Artificial intelligence also has difficulties with planning tasks, especially in stochastic contexts where uncertainty or unpredictability affects results. There is no standard method for the several sorts of inference that researchers employ for planning. It shows that planning itself may be considered a special kind of probabilistic inference and suggests a system for evaluating various inference methods according to how well they manage planning.
Connecting the world’s AI community
Google DeepMind is honored to be a Diamond Sponsor of the conference and to help Black in AI, LatinX in AI, and Women in Machine Learning create communities in data science, machine learning, and artificial intelligence globally.